Toward Distribution Estimation under Local Differential Privacy with Small Samples
Authors: Takao Murakami (National Institute of Advanced Industrial Science and Technology (AIST)), Hideitsu Hino (University of Tsukuba / RIKEN Center for AIP), Jun Sakuma (University of Tsukuba / RIKEN Center for AIP)
Volume: 2018
Issue: 3
Pages: 84–104
DOI: https://doi.org/10.1515/popets-2018-0022
Abstract: A number of studies have recently been made on discrete distribution estimation in the local model, in which users obfuscate their personal data (e.g., location, response in a survey) by themselves and a data collector estimates a distribution of the original personal data from the obfuscated data. Unlike the centralized model, in which a trusted database administrator can access all users’ personal data, the local model does not suffer from the risk of data leakage. A representative privacy metric in this model is LDP (Local Differential Privacy), which controls the amount of information leakage by a parameter called privacy budget. When is small, a large amount of noise is added to the personal data, and therefore users’ privacy is strongly protected. However, when the number of users N is small (e.g., a small-scale enterprise may not be able to collect large samples) or when most users adopt a small value of , the estimation of the distribution becomes a very challenging task. The goal of this paper is to accurately estimate the distribution in the cases explained above. To achieve this goal, we focus on the EM (Expectation-Maximization) reconstruction method, which is a state-of-the-art statistical inference method, and propose a method to correct its estimation error (i.e., difference between the estimate and the true value) using the theory of Rilstone et al. We prove that the proposed method reduces the MSE (Mean Square Error) under some assumptions. We also evaluate the proposed method using three largescale datasets, two of which contain location data while the other contains census data. The results show that the proposed method significantly outperforms the EM reconstruction method in all of the datasets when N or is small.
Keywords: Data privacy, Location privacy, Local differential privacy, EM reconstruction method
Copyright in PoPETs articles are held by their authors. This article is published under a Creative Commons Attribution-NonCommercial-NoDerivs 3.0 license.
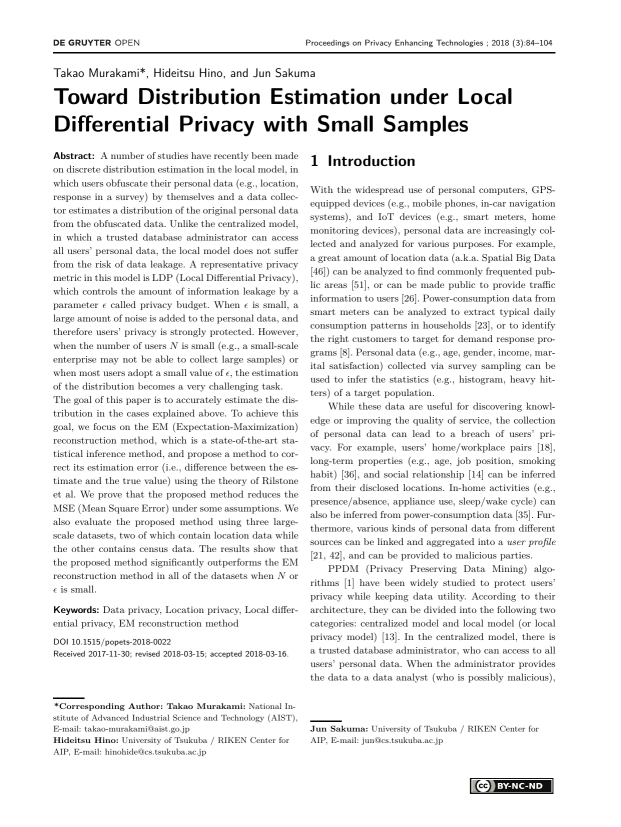