Privacy-Preserving Federated Recurrent Neural Networks
Authors: Sinem Sav (EPFL), Abdulrahman Diaa (University of Waterloo), Apostolos Pyrgelis (EPFL), Jean-Philippe Bossuat (Tune Insight SA), Jean-Pierre Hubaux (EPFL and Tune Insight SA)
Volume: 2023
Issue: 4
Pages: 500–521
DOI: https://doi.org/10.56553/popets-2023-0122
Abstract: We present RHODE, a novel system that enables privacy-preserving training of and prediction on Recurrent Neural Networks (RNNs) in a cross-silo federated learning setting by relying on multiparty homomorphic encryption. RHODE preserves the confidentiality of the training data, the model, and the prediction data; and it mitigates federated learning attacks that target the gradients under a passive-adversary threat model. We propose a packing scheme, multi-dimensional packing, for a better utilization of Single Instruction, Multiple Data (SIMD) operations under encryption. With multi-dimensional packing, RHODE enables the efficient processing, in parallel, of a batch of samples. To avoid the exploding gradients problem, RHODE provides several clipping approximations for performing gradient clipping under encryption. We experimentally show that the model performance with RHODE remains similar to non-secure solutions both for homogeneous and heterogeneous data distributions among the data holders. Our experimental evaluation shows that RHODE scales linearly with the number of data holders and the number of timesteps, sub-linearly and sub-quadratically with the number of features and the number of hidden units of RNNs, respectively. To the best of our knowledge, RHODE is the first system that provides the building blocks for the training of RNNs and its variants, under encryption in a federated learning setting.
Keywords: privacy-preserving machine learning, recurrent neural networks, federated learning, multiparty homomorphic encryption
Copyright in PoPETs articles are held by their authors. This article is published under a Creative Commons Attribution 4.0 license.
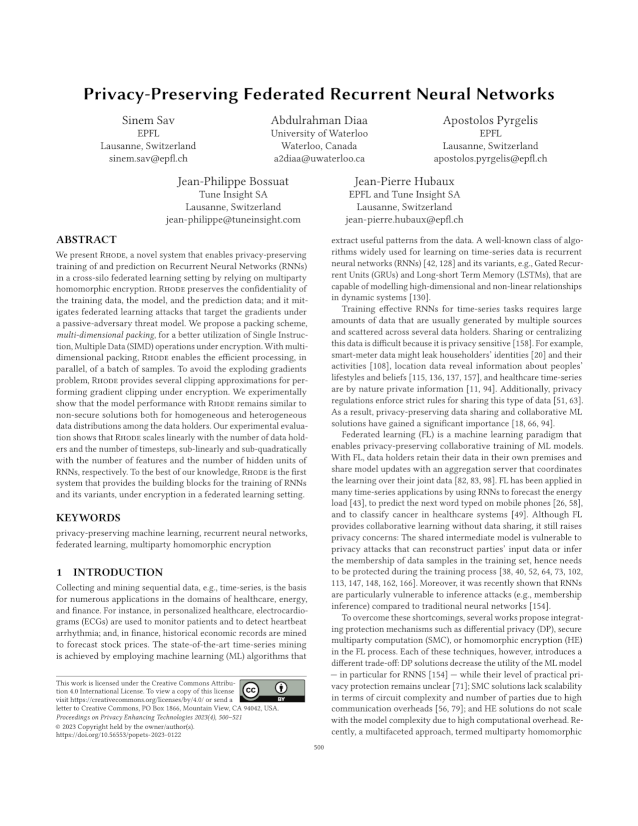