StyleAdv: A Usable Privacy Framework Against Facial Recognition with Adversarial Image Editing
Authors: Minh-Ha Le (Linköping University, Linköping, Sweden), Niklas Carlsson (Linköping University, Linköping, Sweden)
Volume: 2024
Issue: 2
Pages: 106–123
DOI: https://doi.org/10.56553/popets-2024-0043
Abstract: In this era of ubiquitous surveillance and online presence, protecting facial privacy has become a critical concern for individuals and society as a whole. Adversarial attacks have emerged as a promising solution to this problem, but current methods are limited in quality or are impractical for sensitive domains such as facial editing. This paper presents a novel adversarial image editing framework called StyleAdv, which leverages StyleGAN's latent spaces to generate powerful adversarial images, providing an effective tool against facial recognition systems. StyleAdv achieves high success rates by employing meaningful facial editing with StyleGAN while maintaining image quality, addressing a challenge faced by existing methods. To do so, the comprehensive framework integrates semantic editing, adversarial attacks, and face recognition systems, providing a cohesive and robust tool for privacy protection. We also introduce the ``residual attack`` strategy, using residual information to enhance attack success rates. Our evaluation offers insights into effective editing, discussing tradeoffs in latent spaces, optimal edits for our optimizer, and the impact of utilizing residual information. Our approach is transferable to state-of-the-art facial recognition systems, making it a versatile tool for privacy protection. In addition, we provide a user-friendly interface with multiple editing options to help users create effective adversarial images. Extensive experiments are used to provide insights and demonstrate that StyleAdv outperforms state-of-the-art methods in terms of both attack success rate and image quality. By providing a versatile tool for generating high-quality adversarial samples, StyleAdv can be used both to enhance individual users' privacy and to stimulate advances in adversarial attack and defense research.
Keywords: Adversarial samples, Privacy filter, Facial anonymization
Copyright in PoPETs articles are held by their authors. This article is published under a Creative Commons Attribution 4.0 license.
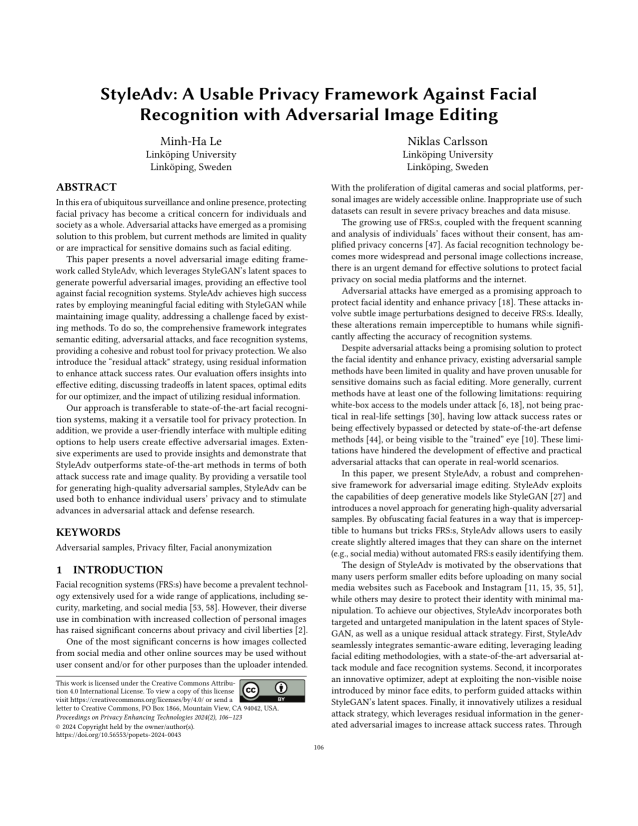