WaKA: Data Attribution using K-Nearest Neighbors and Membership Privacy Principles
Authors: Patrick Mesana (HEC Montréal), Clément Bénesse (University du Québec à Montréal), Hadrien Lautraite (University du Québec à Montréal), Gilles Caporossi (HEC Montréal), Sébastien Gambs (University du Québec à Montréal)
Volume: 2025
Issue: 3
Pages: 494–526
DOI: https://doi.org/10.56553/popets-2025-0110
Abstract: In this paper, we introduce WaKA (Wasserstein K-nearest neighbors Attribution), a novel attribution method that leverages principles from the LiRA (Likelihood Ratio Attack) framework and k-nearest neighbors classifiers (k-NN). WaKA efficiently measures the contribution of individual data points to the model’s loss distribution, analyzing every possible k-NN that can be constructed using the training set, without requiring to sample subsets of the training set. WaKA is versatile and can be used a posteriori as a membership inference attack (MIA) to assess privacy risks or a priori for privacy influence measurement and data valuation. Thus, WaKA can be seen as bridging the gap between data attribution and membership inference attack (MIA) by providing a unified framework to distinguish between a data point’s value and its privacy risk. For instance, we have shown that self-attribution values are more strongly correlated with the attack success rate than the contribution of a point to the model generalization. WaKA’s different usages were also evaluated across diverse real-world datasets, demonstrating performance very close to LiRA when used as an MIA on k-NN classifiers, but with greater computational efficiency. Additionally, WaKA shows greater robustness than Shapley Values for data minimization tasks (removal or addition) on imbalanced datasets.
Keywords: Privacy, K-Nearest Neighbours, Data Attribution, Membership Inference Attack, Data Minimization
Copyright in PoPETs articles are held by their authors. This article is published under a Creative Commons Attribution 4.0 license.
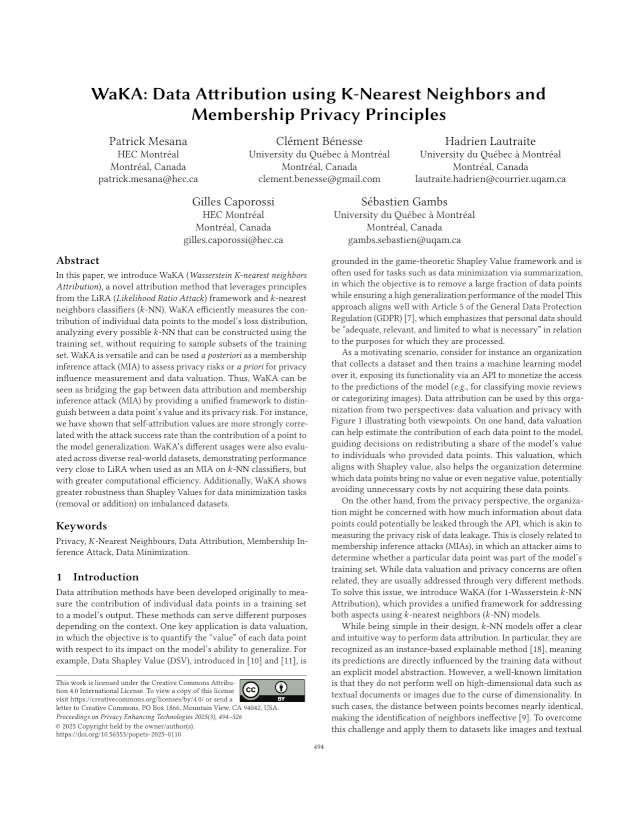